Floyd B. Hanson
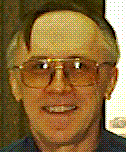
Annotated Publications in
Stochastic Modeling in Biology and Medicine
(Most recent papers have preprint links.)
Some of our important results concern stochastic modeling for biological
and medical applications, many using computational stochastic dynamic
programming, and much of it has been supported by
a series of National Science Foundation Awards (See acknowledgements
below). Preprints of most recent papers, marked by highlighted titles,
are downloadable by clicking on the highlighted title.
13. F. B. Hanson and H. C. Tuckwell,
Persistence Times of Populations with Large Random
Fluctuations, Theor. Population Biol., vol. 14,
pp. 46-61, 1978.
16. C. Tier and F. B. Hanson,
Persistence of Density Dependent Stochastic Populations,
Math. Biosci., vol. 53, pp. 89-117, 1981.
17. F. B. Hanson and H. C. Tuckwell,
Logistic Growth with Random Density Independent Disasters,
Theor. Population Biol., vol. 19, pp. 1-18, 1981.
18. F. B. Hanson and C. Tier,
A Stochastic Model of Tumor Growth,
Math. Biosci., vol. 61, pp. 73-100, 1982.
20. F. B. Hanson and C. Tier,
Harvesting Under Small Demographic Uncertainty,
Lecture Notes in Biomathematics 52, pp. 401-406, 1983.
21. F. B. Hanson and H. C. Tuckwell,
Diffusion Approximation for Neuronal Activity Including Reversal
Potentials,
J. Theor. Neurobiol., vol. 2, pp. 127-153, 1983.
22. D. Ryan and F. B. Hanson,
Optimal Harvesting in Disastrous Environments, in
Mathematical Models of Renewable Resources, vol. II,
edited by R. Lamberson, The Humboldt State
University Mathematical Modeling Group,
Arcata, Cal., pp. 171-177, 1984.
23. D. Ryan and F. B. Hanson,
Poisson search and harvesting, in
Mathematical Models of Renewable Resources, vol. III,
edited by R. Lamberson, The
Humboldt State University Mathematical Modeling Group, Arcata,
Cal., pp. 109-112, 1984.
24. F. B. Hanson and D. Ryan,
Optimal Harvesting in a Stochastic Environment with Density Dependent
Jumps, in
Mathematical Models of Renewable Resources, vol. III,
edited by R. Lamberson, The Humboldt State
University Mathematical Modeling Group, Arcata, Cal., pp. 117-123, 1984.
25. D. Ryan and F. B. Hanson,
Optimal Harvesting with Exponential Growth in an Environment with
Random Disasters and Bonanzas,
Math. Biosci., vol. 74, pp. 37-57, 1985.
26. D. Ryan and F. B. Hanson,
Optimal Harvesting of a Logistic Population in an Environment
with Stochastic Jumps, J. Math. Biol., vol. 24, pp. 259-277,
1986.
29. F. B. Hanson,
Bioeconomic model of the Lake Michigan alewife fishery,
Can. J. Fish. Aquat. Sci., vol. 44, suppl. 2, pp. 298-305,
1987.
Abstract:
This multidimensional fisheries model was developed for the Great Lakes
Fisheries Commission's Assessment and Prediction of Yields (ASPY) Workshop
at Quetico. The model was the motivation for developing high performance
computational algorithms for multidimensional stochastic dynamic programming
solutions.
30[NRM88]. F. B. Hanson and D. Ryan,
Optimal harvesting with density dependent random effects,
Natural Resource Modeling, vol. 2, pp. 439-455, 1988.
Abstract:
This paper was presented at the Third Pacific Coast Resource Modeling
Conference, University of California, Davis, CA, June 1984. The effect of
sudden large, randomly occurring density dependent disasters on the optimal
harvest policy and optimal expected return for an exploited population. The
population grows logistically with disasters occurring on a time scale very
short compared to the natural growth scale. The case of density dependent
frequency is also treated. Stochastic dynamic programming is used in the
optimization and a refined numerical method is successful in computing the
solutions. For a set of realistic field data it is found that random
effects typically have a significant effect on both optimal return and optimal
effort levels. The effect of density dependence is far more pronounced for
optimal return than for optimal effort levels.
35[MBS89]. F. B. Hanson and D. Ryan,
Mean and Quasi-Deterministic Equivalence for Linear Stochastic
Dynamics, Math. Biosci., vol. 93, pp. 1-14, 1989.
Abstract:
In this paper, Hanson and Ryan show an equivalence principle that the
size of the quasi-deterministic population (i.e., using the
infinitesimal mean and variance) is the same as the mean
population governed by a linear, Markov stochastic differential
equation. A new, nonlinear, exactly analyzable, stochastic differential
equation with nontrivial Poisson noise is presented as a counter-example
to show that the linear result is a sharp result.
The importance of this result is that it shows the circumstances
under which the simpler quasi-deterministic approximation can be
used properly in applications.
63[JMB97]. F. B. Hanson and H. C. Tuckwell,
Population Growth with Randomly Distributed Jumps,
J. Math. Biology, vol. 36(2), pp. 169-187, December 1997.
Abstract:
The growth of populations with continuous deterministic and random jump
components is treated.
Three special models in which random jumps occur at the time of
events of a Poisson process and admit formal explicit solutions are
considered:
A) Logistic growth with random disasters having exponentially
distributed amplitudes;
B) Logistic growth with random disasters causing
the removal of a uniformly distributed fraction of the population
size; and C) Exponential decay with sudden increases (bonanzas)
in the population and with each increase being an exponentially
distributed fraction of the current population. Asymptotic and
numerical methods are employed to determine the mean extinction
time for the population, qualitatively and quantitatively.
For Model~A, this time becomes exponentially large
as the carrying capacity becomes much larger than the mean
disaster size. Implications for colonizing species for Model~A are
discussed.
For Model~B and C, the practical notion of
a small, but positive, effective extinction level is chosen, and in
these
cases the expected extinction time rises rapidly with population size,
yet
at less than an exponentially large order.
64[MBS98]. F. B. Hanson and D. Ryan,
Optimal Harvesting with Both Population and Price Dynamics,
Mathematical BioSciences, vol. 148, issue 2, pp. 129-146, March 1998.
Abstract:
We consider the effects of large inflationary price fluctuations
on the computed optimal harvest strategy for a randomized Schaefer model.
Both prices and population sizes are assumed random with both background
(Wiener) and jump (Poisson) components.
Population fluctuations are assumed to be density independent,
i.e., relative changes are independent of population size.
Stochastic dynamic programming is employed to find the
optimal harvesting effort and economic return for a realistic set of
bioeconomic data for Pacific halibut. It is found that inflationary effects
have a pronounced influence on the optimal return, even in a
hazardous or disastrous environment. However, optimal harvesting effort
levels are much less sensitive to inflationary effects.
81[KU02cancer].
J. J. Westman, B. R. Fabijonas, D. L. Kern, and F. B. Hanson,
Probabilistic Rate Compartment Cancer Model:
Alternate versus Traditional Chemotherapy Scheduling,
in Stochastic Theory and Control, Proceedings of a Workshop held
in Lawrence, Kansas, October 18-20, 2001, Lecture Notes in Control and
Information Sciences, B.Pasik-Duncan (Editor), Springer-Verlag,
New York, pp. 491-506, 24 July 2002 (invited paper).
Abstract:
A four-compartment model for the evolution of cancer based on the
characteristics of the cells is presented. The model is expanded to
account for intrinsic and acquired drug resistance. This model can be
explored to see the evolution of drug resistance starting from a single
cell. Numerical studies are performed illustrating the palliative
nature of chemotherapeutic treatments. Computational results are given
for traditional treatment schedules. An alternate schedule for
treatments is developed increasing the life expectancy and quality of
life for the patient. A framework for the alternate scheduling is
presented that addresses life expectancy, quality of life, and risk of
metastasis. A key feature of the alternate schedule is that
information for a particular patient can be used resulting in a
personalized schedule of treatments. Alternate scheduling is compared
to traditional treatment scheduling.
82[MTNS02].
J. J. Westman, t. R. Fabijonas, D. L. Kern, and F. B. Hanson,
Cancer Treatment Using Multiple Chemotherapeutic
Agents Subject to Drug Resistance, Proceedings of the 15th
International Symposium on Mathematical Theory of Networks and Systems,
6 CD pages, 12 August 2002.
Abstract:
A compartment model for the evolution of cancer subject to multiple
chemotherapeutic agents is presented. The formulation accounts for the
heterogeneous nature of cancer and drug resistance, leading to a system
that can be used as a tool for optimizing treatments. Chemotherapeutic
or cytotoxic agents have limited effectiveness due to of toxicity and
the development of drug resistance. Toxicity limits the amount of the
agent that can be used and acts as an upper bound on the dosage. Drug
resistance causes the clinical value of the cytotoxic agent to become
nominal after a small number of treatments. Therefore, several
non-cross resistant cytotoxic agents are used to extend the number of
treatments that can be adminis tered.
93[ACC05]
Siddhartha P. Chakrabarty and Floyd B. Hanson,
Optimal Control of Drug Delivery to Brain Tumors for a
Distributed Parameters Model,
Proceedings of 2005 American Control Conference,
pp. 973-978, 08 June 2005.
Abstract:
The growth and treatment of brain tumors is mathematically examined
using a distributed parameters model. The model is a system of three
coupled reaction diffusion equations involving the tumor cells,
normal tissue and the drug concentration. An optimal control problem
is designed, with the drug delivery rate as the control and solved
to obtain the state and co-state equations as well as the regular
control using a modified double shot forward-backward method.
This gives rise to a
coupled system of equations with a forward state equation and a
backward co-state equation, which is solved using a double shot method.
A numerical procedure based upon the Crank-Nicolson method is
used to solve the coupled system of two three-dimension partial
differential equations.
94[CDC05]
Floyd B. Hanson and Siddhartha P. Chakrabarty,
Optimal Control of Drug Delivery to Brain Tumors for a
PDE Driven Model Using the Galerkin Finite Element Method
,
Proceedings of Joint 44th IEEE Conference on Decision
and Control and European Control Conference,
pp. 1613-1618, 12 December 2005.
Abstract:
The Galerkin finite element method is used to examine the optimal
drug delivery to brain tumors. The PDE driven mathematical model is
a system of three coupled reaction diffusion equations involving the
tumor cells, the normal tissue and the drug concentration. An optimal
control problem is formulated keeping in mind the primary goals of the
treatment, i.e., minimizing the tumor cell density and reducing the side
effects of drugs. A distributed parameter method based on application
of variational calculus to a pseudo-Hamiltonian, is used to obtain a
coupled system of forward state equations and backward co-state equations.
The Galerkin form of the finite element method is used due to its greater
facility in numerically representing complex structures such as those
in the brain. Finally, a two-dimensional circular disk test case is
considered and partitioned into a set of rectangular finite elements in
polar coordinates, with bilinear basis functions in the interior, but
linear-quadratic basis function for elements adjacent to the boundary
to exactly satisfy the no-flux boundary conditions.
98[CDC06]
Siddhartha P. Chakrabarty
and
Floyd B. Hanson,
Cancer Drug Delivery in Three Dimensions For a
Distributed Parameter Control Model Using Finite Elements
,
Proceedings of 45th Conference on Decision and Control
, pp. 2088-2093, San Diego, CA, December 2006.
Abstract:
Optimal distributed parameter control and a Galerkin finite element
method are used to develop procedures for an ideal model of optimal drug
delivery to brain tumors. The mathematical model comprises of a system
of three coupled reaction diffusion models, involving the density of
tumor cells, normal tissue and also the drug concentration. An optimal
control problem is formulated with the goal of minimizing the tumor cell
density and reducing the side effects of the drug. A distributed
parameter method based on a simple application of variational calculus
is used on a pseudo-Hamiltonian, which is then used to obtain a coupled
system of forward state equations and backward co-state equations. The
Galerkin finite element method is used to realistically represent the
brain structure. Finally, an ideal three dimensional test case is
considered and partitioned into a set of brick finite elements in
spherical coordinates, with tri-linear basis functions. Non-uniqueness
of nodes in spherical coordinates is removed by combining like nodes,
such as at the origin, at the poles and at the polar angle
discontinuity. The Galerkin ODEs are solved by a combination of
Crank-Nicolson and predictor-corrector methods.
101[MBS09].
Siddhartha P. Chakrabarty
and
Floyd B. Hanson,
"Distributed Parameters Deterministic Model for Treatment of
Brain Tumors Using Galerkin Finite Element Method
,"
Mathematical Biosciences, pp. 1-54,
accepted for publication, 27 March 2009.
Abstract:
In this paper we present a distributed parameters deterministic model
for
treatment of brain tumors using Galerkin finite element method. The
dynamic
model comprises of system of three coupled reaction diffusion models,
involving the tumor cells, the normal tissues and the drug
concentration.
An optimal control problem is formulated
with the goal of minimizing the tumor cell density and reducing the
side effects of the drug. A distributed parameters method based on
the application of variational calculus is used on an
integral-Hamiltonian,
which is then used to obtain an optimal
coupled system of forward state equations
and backward co-state equations. The Galerkin finite element method is
used to
realistically represent the brain structure as well as to facilitate
computation. Finally a three dimensional test case
is considered and partitioned into a set of
spherical finite elements, using tri-linear basis functions, except for
the
elements effected by singularities of polar and azimuthal angles, as
well the
origin.
Acknowledgements
-
This material is based upon work supported by awards from the National
Science
Foundation under Grants Numbers NSF 880699, 9102343, 9301107, 9626692,
9973231.
-
Supercomputing research support was provided by
- San Diego Supercomputing Center NPACI Account UIL203;
-
National Center for Supercomputing Applications
Grant Numbers DCR860001N, DMS890009N, DMS900016N, DMS920003N,
DMS960002N;
- Pittsburgh Supercomputing Center Grant Numbers DMS940001P and
DMS9400011P;
- Argonne National Laboratory's Advanced Computing Research Facility;
- Los Alamos National Laboratory's Advanced Computing Laboratory
ACL-Accounts Z803835 and gwqfbh-d.
-
Any opinions, findings, and conclusions or recommendations expressed in
this material are those of the author(s) and do not necessarily reflect
the views of the National Science Foundation or other agency.
Web Source: http://www.math.uic.edu/~hanson/stoch-model.html
Email Comments or Questions to Professor Hanson
Back to the
Hanson's CV Page?
Back to the
Hanson's Home Page?
June 1, 1998