Memory Coalescing Techniques¶
To take full advantage of the high memory bandwidth of the GPU, the reading from global memory must also run in parallel. We consider memory coalescing techniques to organize the execution of load instructions by a warp.
Memory Coalescing Techniques¶
Consider two ways of accessing the elements in a matrix: * elements are accessed row after row; or * elements are accessed column after column.
These two ways are shown in Fig. 109.
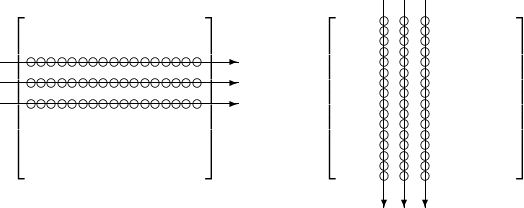
Fig. 109 Two ways of accessing elements in a matrix.
Recall the linear address system to store a matrix. In C, the matrix is stored row wise as a one dimensional array, see Fig. 90.
Threads \(t_0, t_1, t_2\), and \(t_3\) access the elements on the first two columns, as shown in Fig. 110.
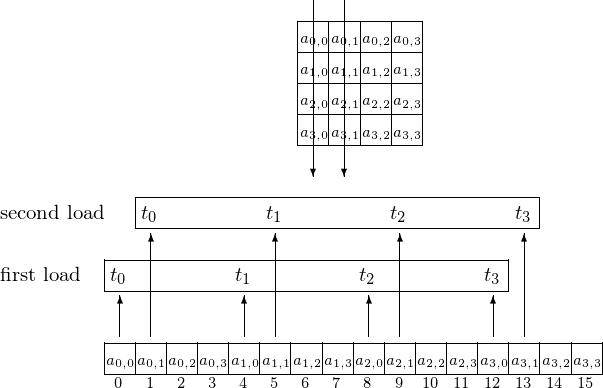
Fig. 110 Accessing elements column after column.
Four threads \(t_0, t_1, t_2\), and \(t_3\) access elements on the first two rows, as shown in Fig. 111.
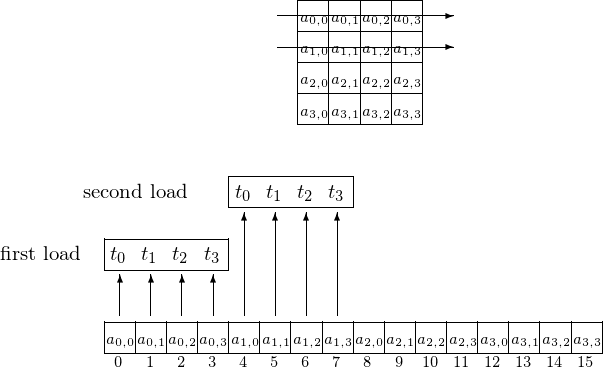
Fig. 111 Accesing elements row after row.
The differences between uncoalesced and coalesced memory accesses are shown in Fig. 112.
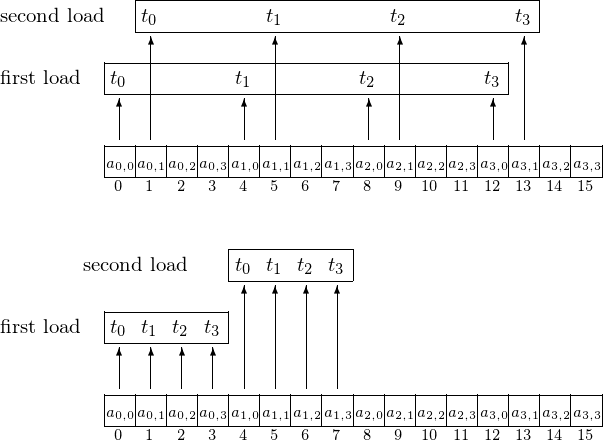
Fig. 112 Uncoalesced versus coalesced access.
We can use shared memory for coalescing. Consider Fig. 99 for the tiled matrix-matrix multiplication.
For \(\displaystyle C_{i,j} = \sum_{k=1}^{m/w} A_{i,k} \cdot B_{k,j}\), \(A \in {\mathbb R}^{n \times m}\), \(B \in {\mathbb R}^{m \times p}\), \(A_{i,k}, B_{k,j}, C_{i,j} \in {\mathbb R}^{w \times w}\), every warp reads one tile \(A_{i,k}\) of \(A\) and one tile \(B_{k,j}\) of \(B\): every thread in the warp reads one element of \(A_{i,k}\) and one element of \(B_{k,j}\).
The number of threads equals w, the width of one tile, and threads
are identified with tx = threadIdx.x
and ty = threadIdx.y
.
The by = blockIdx.y
and bx = blockIdx.x
correspond
respectively to the first and the second index of each tile, so we have
row = by*
w + ty
and col = bx*
w + tx
.
Row wise access to A uses A [row*m + (k*w + tx)]
.
For B: B [(k*w+ty)*m + col]
= B [(k*w+ty)*m + bx*w+tx]
.
Adjacent threads in a warp have adjacent tx
values
so we have coalesced access also to B.
The tiled matrix multiplication kernel is below:
__global__ void mul ( float *A, float *B, float *C, int m )
{
__shared__ float As[w][w];
__shared__ float Bs[w][w];
int bx = blockIdx.x; int by = blockIdx.y;
int tx = threadIdx.x; int ty = threadIdx.y;
int col = bx*w + tx; int row = by*w + ty;
float Cv = 0.0;
for(int k=0; k<m/w; k++)
{
As[ty][tx] = A[row*m + (k*w + tx)];
Bs[ty][tx] = B[(k*w + ty)*m + col];
__syncthreads();
for(int ell=0; ell<w; ell++)
Cv += As[ty][ell]*Bs[ell][tx];
C[row][col] = Cv;
}
}
Avoiding Bank Conflicts¶
Consider the following problem:
On input are \(x_0, x_1, x_2, \ldots x_{31}\), all of type float.
The output is
This gives 32 threads in a warp 1,024 multiplications to do. Assume the input and output resides in shared memory. How to compute without bank conflicts?
Suppose we observe the order of the output sequence. If thread \(i\) computes \(x_i^2, x_i^3, x_i^4, \ldots, x_i^{33}\), then after the first step, all threads write \(x_0^2, x_1^2, x_2^2, \ldots, x_{31}^2\) to shared memory. If the stride is 32, all threads write into the same bank. Instead of a simultaneous computation of 32 powers at once, the writing to shared memory will be serialized.
Suppose we alter the order in the output sequence.
After the first step, thread \(i\) writes \(x_i^2\) in adjacent memory, next to \(x_{i-1}^2\) (if \(i > 0\)) and \(x_{i+1}^2\) (if \(i < 31\)). Without bank conflicts, the speedup will be close to 32.
Exercises¶
- Run
copyKernel
for large enough arrays for zerooffset
and anoffset
equal to two. Measure the timings and deduce the differences in memory bandwidth between the two different values foroffset
. - Consider the kernel of
matrixMul
in the GPU computing SDK. Is the loading of the tiles into shared memory coalesced? Justify your answer. - Write a CUDA program for the computation of consecutive powers, using coalesced access of the values for the input elements. Compare the two orders of storing the output sequence in shared memory: once with and once without bank conflicts.